March Madness Results: The Tale of A Busted Bracket
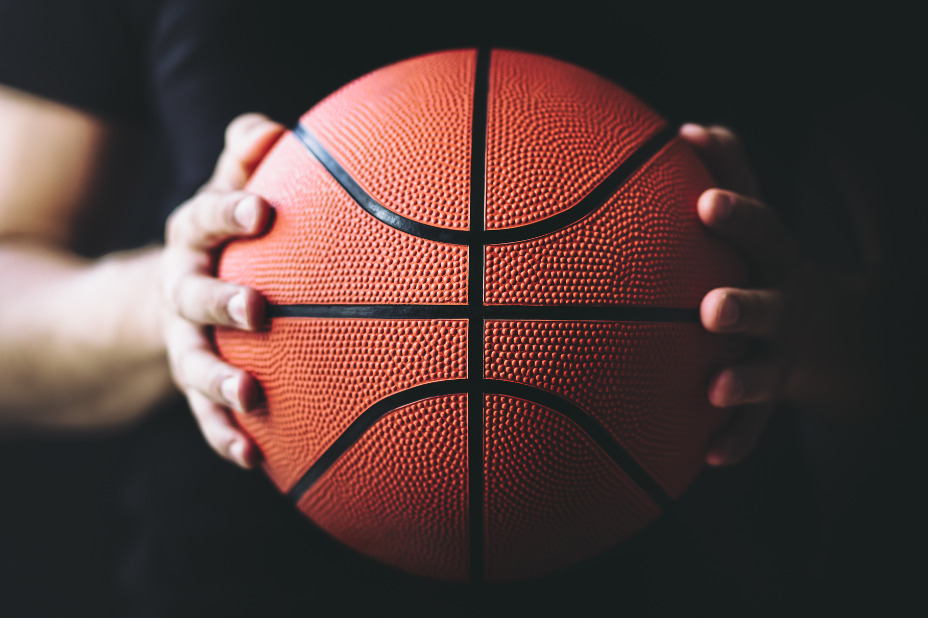
With the release of the official March Madness Results, our Flatiron School Machine Learning bracket was busted. Here’s how it happened.
After 3 weeks and 67 games, March Madness ended with disappointment for most fans. While you may still be recovering from the gray-hair-inducing stress-fest that is the annual tournament (we recommend ripping up your paper bracket – it’s very cathartic), it’s a good time to look back at where we started and how things went so, very wrong for the official Flatiron School bracket.
Our Machine Learning Bracket Prediction
In March, Data Science Curriculum Developer Brendan Purdy used Machine Learning to develop a March Madness bracket, which you can see below. Visit this blog post to learn how he used Machine Learning to develop his March Madness bracket.
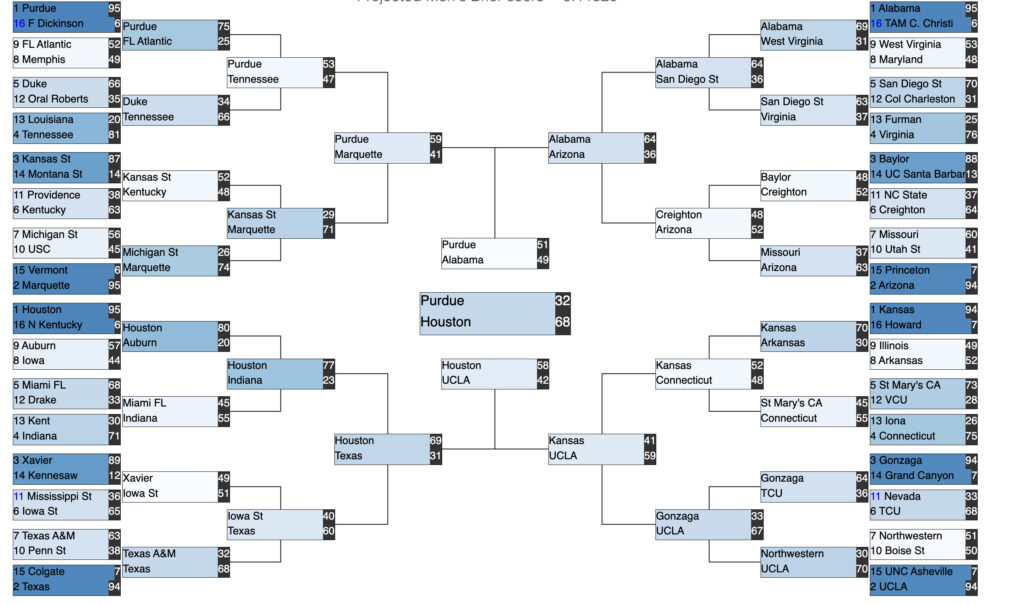
Unfortunately, the Machine Learning generated bracket did not perform well. Purdy’s bracket correctly predicted only 2 of the final 8 teams and none of the final 4.
March Madness Results
This year’s bracket had quite a few surprises, with favorite teams like UCLA and Purdue not even making it to the final 8. And with San Diego beginning at a 25.7% win probability, it shocked many that they made it all the way to the National Championship.

For a team-by-team breakdown of each defeat and unexpected upset, visit ESPN’s March Madness Results Pain Scale and be comforted by the fact that your agony is shared.
So, What Happened?
First off, let’s put some numbers into perspective around the March Madness Results. There are 9,223,372,036,854,775,808 possible outcomes for a bracket, so you’re more likely to win the lottery (or several lotteries) than guess a perfect bracket. And despite the more than 70 official brackets submitted each year, the longest (verifiable) streak of an NCAA men’s bracket ever was only 49 games, where the person predicted all of the teams who got into the Sweet 16 in 2022.
So, whether Machine Learning and AI are used to generate a bracket or not, the odds are slim.
Machine Learning Constraints
Data Sets and Inputs
The algorithm uses certain assumptions to generate outputs based on provided inputs, and so makes predictions based on data trends. So, if team A has consistently beaten B, then there is a high probability that they’ll do it again, and that is what the AI will predict.
Where the training data is obtained from and the different weights they attribute to ranking factors such as historical seeding, performance (both season and postseason), box scores, geography, coaches, etc. can greatly impact the linear regression model’s predictions.
Preprocessing/ Feature Engineering
The preprocessing or feature engineering stage of creating a Machine Learning model is one of the most challenging steps. This requires bringing disparate data sets together, getting the variables in the proper form so that we can use the algorithm, and other cleaning of the data to focus the model on certain variables.
Naturally, this can result in varied inputs and thus varied outputs. If fact, two Data Scientists given the same data set will inevitably preprocess it in slightly different ways, leading to distinct results.
Dumb Luck
No matter how perfectly ranked your stats are, how precisely programmed the data set is, or the number of iterations your model runs, there are certain things a Machine Learning model won’t be able to account for. The model makes predictions based on previous data and past performance and predicts outcomes based on the same conditions.
So if, for example, a star player is out of the game, the whole team got food poisoning the night before, or a hail mary shot somehow made it through the next in the last second of the game, the model does not expect nor account for random good or bad luck.
Conclusion
As fans can attest, there is no greater torment than watching your bracket inevitably go bust. And, while Machine Learning may increase your chances of hanging in longer, it’s almost inevitable that your bracket predictions will eventually prove incorrect. But if we’re honest, isn’t that half of the fun? From one busted bracket to another – better luck next year.
Wanna try your hand at the Data Science fundamentals needed to make a Machine Learning model like the one discussed in this post? Try out our Free Data Science Prep Work – no strings attached.
Disclaimer: The information in this blog is current as of April 28, 2023. Current policies, offerings, procedures, and programs may differ.